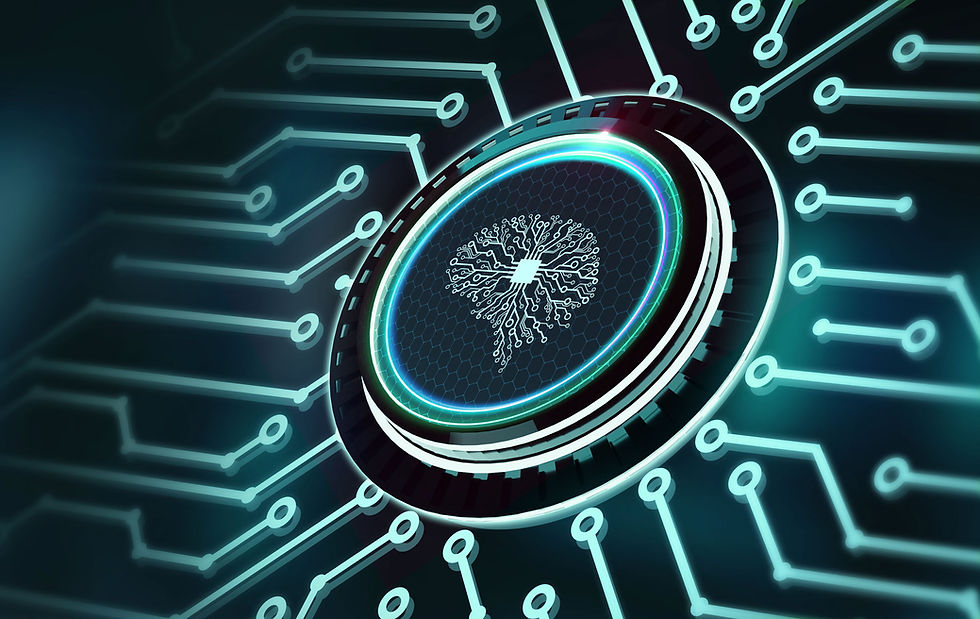
Introduction
Generative systems have revolutionized the creative landscape, introducing unprecedented ways to blend human ingenuity with computational power. Two prominent offshoots of this evolution are generative art and generative AI. While these terms are often used interchangeably, they represent distinct approaches with unique goals and methodologies.
Generative art is a celebration of algorithm-driven aesthetics, where artists define rules and constraints, allowing algorithms to produce captivating visual and auditory experiences. This field emphasizes creativity and collaboration between human intent and computational randomness.
On the other hand, generative AI focuses on harnessing the power of artificial intelligence to create functional outputs such as realistic images, natural language text, and synthesized audio. With applications in industries ranging from entertainment to healthcare, generative AI is reshaping what machines can achieve independently.
In this blog, we’ll delve into the defining characteristics of generative art and generative AI, exploring their similarities, differences, and the profound impact they have on the world of creativity and technology.
What is Generative Art?
Generative art is a form of artistic expression where creativity meets computation. It is defined by the use of algorithms, rules, or systems to create works of art that are often unpredictable and highly unique. While the artist sets the parameters and constraints, the process itself introduces a degree of randomness or automation, resulting in outcomes that even the creator cannot fully anticipate.
Historical Context
Generative art has roots in the mid-20th century, predating modern computing. Early pioneers like Vera Molnar, one of the first to experiment with algorithmic drawings, and Harold Cohen, creator of the AI-driven art program AARON, explored the intersection of art and technology long before digital tools became widespread. These artists sought to expand the boundaries of creativity by introducing mathematical precision and randomness into the artistic process.
Core Principles of Generative Art
At its core, generative art relies on a blend of human intention and computational processes. Artists design algorithms or systems that dictate how the artwork will unfold. Key principles include:
Randomness and Constraints: Controlled randomness allows the creation of unique pieces within predefined rules.
Interactivity: Some generative art evolves in response to user input or environmental factors, such as sound or movement.
Iteration: Repetition and variation within a system generate intricate patterns and structures.
This method of creation challenges traditional notions of authorship, as the final work often emerges as a collaboration between human and machine.
Common Tools and Techniques
Generative art can be created using a wide array of tools and techniques. Some of the most popular include:
Software: Programs like Processing, p5.js, and TouchDesigner enable artists to code visual expressions.
Hardware: Physical installations often involve Arduino boards, sensors, or robotics.
Mathematical Models: Algorithms such as fractals, Perlin noise, and cellular automata are frequently used to generate patterns and forms.
Aesthetic and Emotional Value
The appeal of generative art lies in its ability to surprise and engage. By incorporating randomness into structured systems, it creates works that feel organic and alive. Generative art’s unpredictable nature allows for exploration of themes like chaos, order, and the intersection of human creativity with machine intelligence.
Whether displayed as digital graphics, interactive installations, or even NFT collections, generative art continues to push the boundaries of what is possible when art and technology collide.
What is Generative AI?
Generative AI represents a cutting-edge branch of artificial intelligence, focusing on the creation of new content by leveraging learned patterns from data. Unlike traditional AI systems that perform tasks based on predefined rules, generative AI uses machine learning models to produce outputs such as text, images, music, and even complex code. It operates at the intersection of computation, creativity, and functionality, making it one of the most transformative technologies of our time.
Definition and Core Concepts
Generative AI is fundamentally about creating. It employs advanced algorithms, such as neural networks, to analyze vast datasets and generate new outputs that mimic the style or characteristics of the data it was trained on. For instance, generative AI can create a painting in the style of Van Gogh, write an article, or generate lifelike voices.
Key technologies driving generative AI include:
Generative Adversarial Networks (GANs): Systems where two neural networks—the generator and the discriminator—work in opposition to create highly realistic outputs.
Transformer Models: Algorithms like OpenAI’s GPT (Generative Pre-trained Transformer) and Google’s BERT revolutionized text-based AI applications.
Diffusion Models: Used to generate detailed images, diffusion models reverse noise processes to create visually stunning outputs, as seen in tools like DALL-E and Stable Diffusion.
How Generative AI Works
Generative AI relies on training data to learn patterns, relationships, and structures. The process typically involves three stages:
Data Training: A machine learning model is exposed to a dataset, such as images, text, or audio, and trained to recognize underlying patterns.
Pattern Replication: Using the learned patterns, the AI generates new content that mimics the characteristics of the input data.
Optimization: Feedback loops refine the outputs to ensure quality and relevance, often with human input to guide the process.
For example, a language model like GPT can write a poem by analyzing a vast corpus of poetry and learning the syntax, style, and structure.
Applications of Generative AI
Generative AI’s versatility has led to its adoption across numerous industries:
Text Generation: Tools like ChatGPT can draft articles, answer questions, or even generate code.
Image and Video Creation: Models such as MidJourney and DALL-E produce highly detailed images, while deepfake technology creates realistic videos.
Music and Sound Design: AI-driven tools generate original compositions or adapt existing pieces to new styles.
Product Design: Generative AI helps create innovative designs for everything from fashion to automotive engineering.
Healthcare: Applications include drug discovery through AI-generated molecule designs and personalized medical reports.
A New Paradigm of Creativity
Generative AI blurs the line between human creativity and machine-driven innovation. Unlike generative art, which often prioritizes aesthetics and emotional impact, generative AI is deeply functional, designed to solve problems and optimize processes. Its outputs can be indistinguishable from human-created content, raising questions about originality, authorship, and ethics.
As generative AI continues to evolve, it promises to redefine how we create, interact, and innovate across virtually every domain.
Comparing Generative Art and Generative AI
Generative art and generative AI are both transformative approaches to creativity that harness computational processes, but they serve different purposes, rely on distinct tools, and engage with creativity in unique ways. While they share some common ground in their reliance on algorithms, their goals, methods, and cultural implications set them apart.
Goals and Intentions
Generative Art:The primary goal of generative art is aesthetic and emotional expression. Artists use algorithms to create works that evoke beauty, provoke thought, or communicate complex ideas. It emphasizes artistic intent, often relying on randomness or systems to introduce uniqueness and unpredictability.
Generative AI:Generative AI is often driven by functionality and utility. Its outputs—whether text, images, or other media—are typically designed to solve problems, streamline workflows, or replicate human-like creation. While it can be used for artistic purposes, its overarching goal is usually practical rather than purely artistic.
Tools and Technologies
Generative Art:Tools used in generative art are often tailored to artistic exploration, such as:
Programming languages like Processing or p5.js.
Hardware setups like Arduino for interactive installations.
Techniques involving randomness, fractals, and cellular automata to generate organic patterns.
Generative AI:Generative AI relies on advanced machine learning models, which include:
Generative Adversarial Networks (GANs): For creating realistic images or videos.
Transformer Models: For tasks like text generation (e.g., GPT).
Diffusion Models: For high-quality image generation (e.g., DALL-E, Stable Diffusion).
These tools often require extensive training on large datasets, as opposed to the rule-based systems often seen in generative art.
Role of Human Creativity
Generative Art:Human creativity plays a central role in generative art. The artist determines the rules, constraints, and aesthetic direction of the work. While algorithms execute the process, the artist’s vision and decisions shape the outcome. This collaboration between artist and machine is a hallmark of the field.
Generative AI:Generative AI operates with minimal direct creative input from humans. Once trained, it can produce outputs autonomously, guided only by prompts or user specifications. The human role is often more about refining or curating the results rather than shaping them actively during creation.
Outputs and Applications
Generative Art:The outputs of generative art are typically limited to the realm of visual, auditory, or experiential works designed to be appreciated as art. These outputs are showcased in galleries, interactive installations, or digital platforms like NFT marketplaces.
Generative AI:Generative AI’s applications are vast, ranging from creating chatbots like ChatGPT and image generators like DALL-E to practical uses in healthcare, design, and marketing. Its outputs often prioritize functionality, such as creating usable designs, generating human-like conversations, or synthesizing data insights.
Philosophical Perspectives
Generative Art: Generative art often explores philosophical questions about creativity, randomness, and the role of the artist. The concept of shared authorship between human and machine is central, challenging traditional ideas of originality.
Generative AI: Generative AI raises ethical and philosophical debates around its potential to replace human creativity. Concerns include questions about authorship, bias in AI-generated content, and the implications of AI-driven automation on human roles.
Conclusion
Generative art and generative AI, while interconnected, represent two distinct approaches to computational creativity. Generative art emphasizes artistic expression, relying on human-defined rules and constraints to create visually or emotionally evocative works. It thrives in the realm of unpredictability, celebrating the collaboration between human intention and algorithmic processes. On the other hand, generative AI focuses on functional innovation, employing machine learning to generate practical, often utility-driven outputs like text, images, or sound.
Both fields highlight the transformative potential of algorithms, but their goals, tools, and impacts diverge significantly. Generative art seeks to push the boundaries of creativity, while generative AI often seeks to replicate or augment human capabilities across industries.
Understanding the distinctions between the two helps us appreciate their unique contributions to the creative and technological landscapes. As these fields continue to evolve, they will undoubtedly reshape how we think about art, technology, and the role of humanity in a world increasingly influenced by intelligent systems. Whether inspiring awe through algorithmic beauty or solving real-world problems with artificial intelligence, both generative art and generative AI reflect the power of computation to expand the horizons of possibility.
Comments